Derek DeSantisPostdoc T-3/CNLS Machine Learning, Climate Science, Operator Algebras 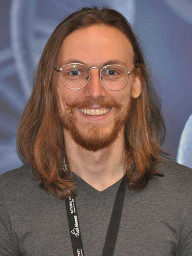
Office: TA-3, Bldg 1690, Room 136 Mail Stop: B258 Phone: (505) 667-9331 Fax: (505) 665-2659 ddesantis@lanl.gov home page Research highlight- Mathematical Theory of Tensor Factorizations
- Unsupervised Machine Learning in Climate Sciences
- Operator Theory and Operator Algebras
|  | Educational Background/Employment:- (2012) B.S. Mathematics, B.S. Physics - California State University - Channel Islands
- (2014) Masters Mathematics - University of Nebraska - Lincoln
- (2019) Ph.D. Mathematics - University of Nebraska - Lincoln
Research Interests: - Mathematical Theory of Tensor Factorizations - Data measured from a high-order complex system can be difficult to analyze. Tensors are a convenient
mathematical object describe data. However, the dependencies between the indices is not clear, making interpretation of the data a demanding task. The main goal of
all tensor factorizations is to decompose the tensor into simpler objects, such as sums special products of matrices. These atomized, simple building blocks often
reflect the hidden processes that went into deriving the data. I work on proving existence and uniqueness theorems for different types of tensor factorizations.
- Unsupervised Machine Learning in Climate Sciences - The Earth's climate system is complex. There are many physical forces that driving components of
the system, many of which are latent. By applying unsupervised machine learning techniques, one can harvest meaningful information hidden in the data. I work on
developing model specific clustering and tensor factorization methods for analyzing different aspects of the Earth's climate system.
- Machine Learning Safety - Rapid progress in machine learning (ML) has engendered numerous applications across the sciences. Deployment of modern
ML systems have increased our ability to validate and automate the scientific process, broadening the space for discovery. However, these advances come with their
own associated challenges and risks. For example, optimization schemes incorporated during training are necessarily opaque, leading one to question if the algorithm
has learned features of nature or artifacts of the data. This is the ML safety problem. A recent trend emerging from these types of issues is the desire to make ML systems more explainable and
interpretable. I work on building model specific as well as model agnostic methods for increasing ML safety.
- Operator Theory and Operator Algebras - The field of operator algebras studies collections of particular types of functions between Hilbert spaces. These
collections often encode other algebraic objects of interest, such as (directed) graphs. I developed the theory behind a class of operator algebras that encodes
the dynamics of Hilbert space frames. I'm currently working on encoding Markov Decision Processes into an operator theoretic framework as well.
Selected Recent Publications:
|